Introduction to Machine Learning in Product Management
In recent years, the integration of machine learning in product management has revolutionized the way companies develop, optimize, and personalize their offerings. By leveraging advanced algorithms and data-driven insights, product managers can make informed decisions that enhance user experiences, drive product innovation, and streamline operations.
Key points:
- Introduction to Machine Learning in Product Management
- Basics of Machine Learning
- Importance of Machine Learning in Product Management
- Incorporating Machine Learning in Product Development
- Overcoming Challenges in Machine Learning Product Management
Understanding the Basics of Machine Learning
Machine learning is a subset of artificial intelligence that focuses on developing algorithms capable of learning from data to make predictions or decisions. Through processes like supervised learning, unsupervised learning, and reinforcement learning, machines can recognize patterns, generate insights, and improve their performance over time.
The Importance of Machine Learning in Product Management
Machine learning plays a pivotal role in product management by enabling teams to extract valuable insights from vast amounts of data, uncovering trends, and user behaviors that guide strategic decisions. By leveraging predictive analytics, companies can forecast demand, personalize offerings, and optimize pricing strategies.
Key Considerations for Implementing Machine Learning in Product Management
When incorporating machine learning into product management processes, it’s crucial to consider factors like data quality, model transparency, and ethical implications. Understanding the limitations and biases inherent in machine learning models is essential for leveraging the technology effectively.
Incorporating Machine Learning in Product Development
To effectively harness the power of machine learning in product management, companies must identify opportunities for integration, develop a comprehensive strategy, and rigorously test and evaluate models.
Identifying Opportunities for Machine Learning Integration
Product managers can pinpoint opportunities for incorporating machine learning by analyzing customer behavior, market trends, and competitive landscapes. By identifying pain points and inefficiencies, teams can leverage machine learning to drive innovation and create differentiated products.
Building a Machine Learning Strategy for Product Development
Developing a robust machine learning strategy involves defining clear objectives, selecting appropriate algorithms, and establishing key performance indicators to measure success. By aligning machine learning initiatives with business goals, companies can maximize the value generated from data-driven insights.
Testing and Evaluating Machine Learning Models in Product Management
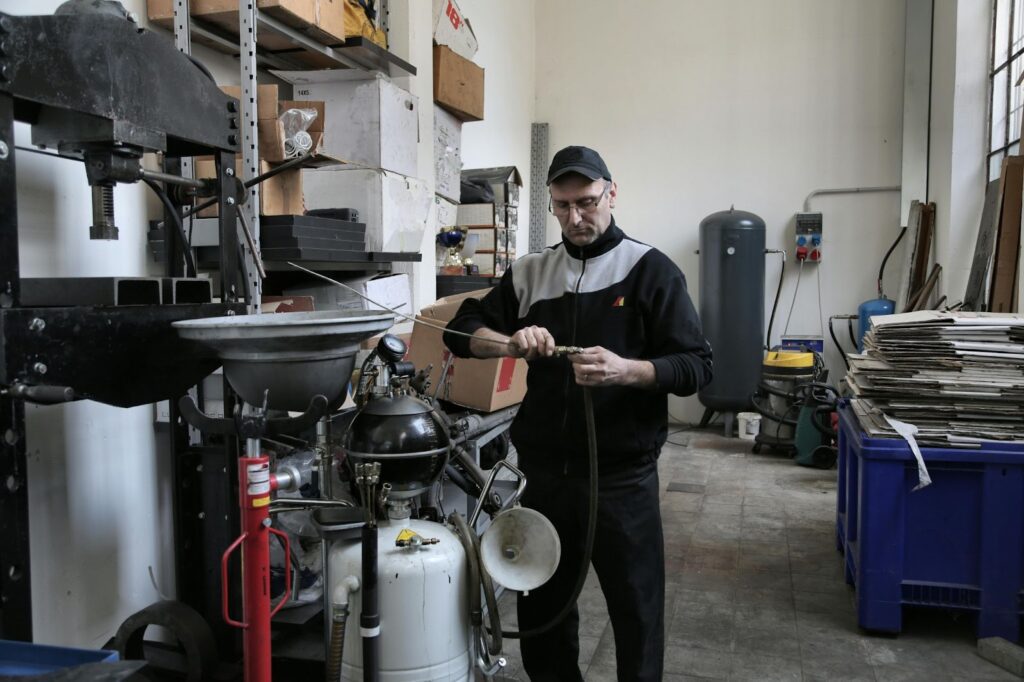
Testing and evaluating machine learning models are critical steps in ensuring their accuracy and effectiveness. By conducting A/B testing, cross-validation, and performance monitoring, product managers can iteratively improve models and optimize their impact on product development.
Improving User Experience with Machine Learning
Personalizing user experiences, enhancing product recommendations, and implementing sentiment analysis are key ways in which machine learning can elevate user satisfaction and drive engagement.
Personalizing User Experiences with Machine Learning
By leveraging machine learning algorithms, companies can deliver personalized content, recommendations, and interactions tailored to individual user preferences. Personalization enhances user engagement, retention, and loyalty, leading to increased customer satisfaction and conversion rates.
Enhancing Product Recommendations through Machine Learning
Product recommendations powered by machine learning algorithms can provide users with relevant, timely suggestions that drive sales and cross-selling opportunities. By analyzing user behavior and purchase history, companies can offer personalized recommendations that increase customer satisfaction and drive revenue growth.
Implementing Sentiment Analysis for Customer Feedback
Sentiment analysis enables product managers to gauge customer sentiment, identify trends, and address issues proactively. By analyzing customer feedback from surveys, reviews, and social media, companies can extract valuable insights that inform product development decisions and improve overall customer satisfaction.
Machine Learning for Product Optimization
From pricing optimization to supply chain management and marketing campaigns, machine learning can optimize product performance across various dimensions.
Utilizing Machine Learning for Pricing Optimization
Machine learning algorithms can analyze pricing data, competitor pricing strategies, and market dynamics to optimize pricing models for maximized profitability. By dynamically adjusting prices based on demand, seasonality, and competitive factors, companies can enhance revenue streams and improve market positioning.
Streamlining Supply Chain Management with Machine Learning
Machine learning can optimize supply chain operations by forecasting demand, optimizing inventory levels, and automating procurement processes. By leveraging predictive analytics, companies can reduce lead times, minimize costs, and enhance supply chain resilience in the face of disruptions.
Improving Marketing Campaigns using Machine Learning
Machine learning enables marketers to create targeted, personalized campaigns that resonate with specific audience segments. By analyzing customer behavior, preferences, and engagement metrics, companies can tailor messaging, offers, and channels to drive higher conversion rates and ROI.
Overcoming Challenges in Machine Learning Product Management
While the benefits of machine learning in product management are significant, companies must address challenges related to data privacy, algorithm bias, and resource allocation to ensure successful implementation.
Addressing Data Privacy and Security Concerns
Ensuring data privacy and security is paramount when leveraging machine learning algorithms that analyze sensitive customer information. By implementing robust data protection measures, encryption protocols, and access controls, companies can safeguard user data and build trust with customers.
Dealing with Algorithm Bias in Product Development
Algorithm bias can lead to unfair or discriminatory outcomes in product management processes, impacting user experiences and brand reputation. To mitigate bias, product managers must audit models, address biases in training data, and prioritize diversity and inclusivity in algorithm development.
Managing Resources and Budget for Machine Learning Initiatives
Effective resource allocation and budgeting are essential for successful machine learning initiatives in product management. By prioritizing high-impact projects, fostering cross-functional collaboration, and investing in talent development, companies can maximize the ROI of their machine learning investments.
Summary
This comprehensive guide has explored the multifaceted role of machine learning in product management, highlighting its benefits, challenges, and best practices. From personalizing user experiences to optimizing products through data-driven insights, integrating machine learning into product development is essential for modern product managers seeking to drive innovation, enhance customer satisfaction, and achieve business growth.
FAQs
What are some common machine learning algorithms used in product management?
Common algorithms include Linear Regression for forecasting, Decision Trees for classification, and Neural Networks for complex pattern recognition tasks.
How can companies ensure ethical machine learning practices in product development?
By using diverse datasets to mitigate biases, conducting regular audits for fairness, and ensuring transparent documentation of algorithms and data usage.
What are the key metrics to track the success of machine learning initiatives in product management?
Metrics such as accuracy (precision, recall), user engagement (click-through rates), and business KPIs (revenue growth, customer retention) provide insights into the effectiveness of machine learning initiatives.